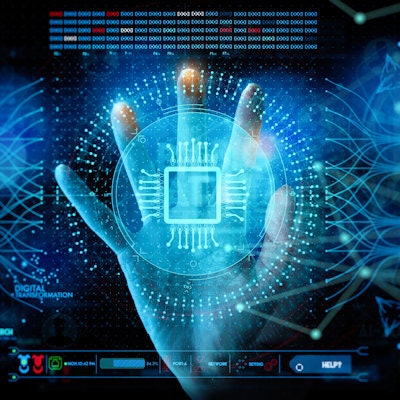
Google scientists reported proof-of-concept data for their deep-learning system in analyzing pathology images and predicting survival of cancer patients in PLOS One on June 17.
The deep-learning system was developed and tested using histopathology slides for patients with 10 cancer types -- including bladder cancer, invasive breast cancer, and head and neck squamous cell carcinoma -- drawing from publicly available data from the Cancer Genome Atlas (TCGA) program.
Training for the system involved 9,086 digitized whole-slide images from 3,664 cases. Testing encompassed 3,009 digitized images from 1,216 cases. Overall, the deep-learning system scoring was significantly associated with disease-specific survival, Google computer scientist Ellery Wulczyn and colleagues reported, noting a hazard ratio of 1.58.
Drilling down to performance by tumor type, the system was associated with significant prediction of survival in five of the 10 cancers studied (p = 0.0002 to p = 0.0257). It was also possible to stratify risk within some cancer stages (II and III).
"Our analysis demonstrates the potential for this approach to provide significant prognostic information in multiple cancer types, and even within specific pathologic stages," Wulczyn and colleagues wrote.
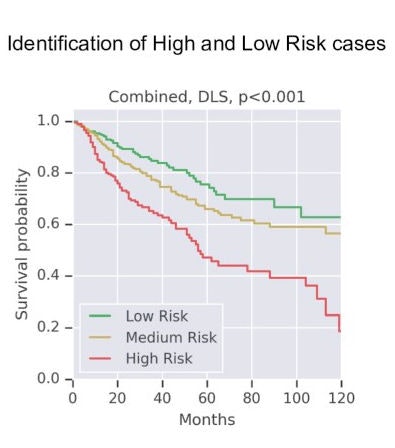
An important limitation of the study was that even though the researchers drew upon the TCGA, which they noted is the largest publicly available dataset, they had limited numbers of cases and disease-specific survival events for each tumor type (fewer than 250 cases and 100 events).
Consequently, they reported wide confidence intervals in the performance results and view the data as supporting proof-of-concept but needing a lot more work.
Bolstering risk stratification
Artificial intelligence shows promise for improving the ability to predict survival and risk stratification in cancer patients beyond conventional tools like the TNM Classification of Malignant Tumors system.
"Although TNM staging is useful and well-studied, there is room for improvement in some settings, with ongoing efforts to develop improved prediction strategies that incorporate information such as clinical variables, genetic information, and histomorphological features including tumor grade," Wulczyn and colleagues wrote. "In this regard, computational image analysis of tumor histopathology offers an emerging approach to further improve patient outcome predictions by learning complex and potentially novel tumor features associated with patient survival."
Deep-learning approaches promise automated learning of prognostic features, but a development challenge is that they typically entail the use of large datasets annotated by humans.
"In histopathology, these models are typically trained on millions of small image patches taken from [whole-slide images] of digitized pathology slides that have specific features of interest labeled by pathologists, often involving detailed, hand-drawn annotations," the authors wrote.
The weakly supervised learning system developed by Google is less laborious by comparison. The machine-learning model is given limited information during the training process, Yun Liu, PhD, a senior research scientist at Google Health, explained to LabPulse.com. In this case, the model is told only the information about a certain clinical outcome, disease-specific survival, but is not told what to look for nor where in each gigapixel whole-slide image the pertinent information is. Many small patches from within large histopathology images are sampled and the model then provides an overall predicted prognosis for the case, which Google analysts believe can help predict outcomes for several cancers in the publicly available TCGA dataset.
"While the results reported here provide support for the feasibility of developing weakly supervised deep learning models to predict patient prognosis from whole-slide images across multiple cancer types, future work exploring and validating the potential of deep learning applications for this task will require larger, clinically representative datasets," the authors concluded.
Liu noted that machine-learning tools are complementary to the expertise of healthcare professionals and are best suited to augmenting their work.
"The depth and breadth of expertise that pathologists provide, combined with their ability to understand the nuance and context of every case, provide a critical and remarkable element of the work that machines alone are unlikely to address," he said.