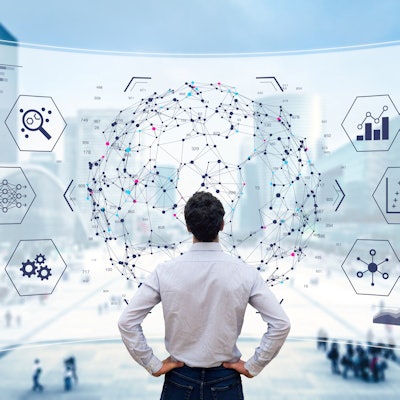
To realize the full potential of artificial intelligence (AI) in diagnostics, it is time to develop a new generation of tools that assist clinicians during a patient's path to diagnosis rather than tools that predict final diagnoses, asserts a viewpoint published on December 28 in JAMA.
"A new generation of AI is needed that considers the dynamism of the diagnostic process and answers the questions of where are the clinician and patient on the diagnostic pathway and what should be done next," wrote the authors, led by Julia Adler-Milstein, PhD, of the department of medicine at the University of California, San Francisco.
In making a diagnosis, clinicians must synthesize complex patient data and determine the best course of action, the authors noted. The entire process, which the authors refer to as "wayfinding," can be enhanced by using AI tools designed to reduce the clinician's cognitive load during the decision-making process.
"AI tools that support the dynamic diagnostic refinement process should help the clinician understand where they are on the diagnostic pathway and help them select the paths most likely to reduce uncertainty," the authors wrote. "The clinician still analyzes data and makes decisions, with AI serving to lower the cognitive load."
The authors describe diagnosis as a "dynamic refinement process" consisting of the following steps, which begin after a patient presents with signs and symptoms:
- Information gathering, organization, and prioritization
- Information integration and interpretation
- Formulation of next steps
- Leading diagnosis
- Final diagnostic label
- Development of care plan
Steps one through three are iterated in a cycle as the diagnosis is refined. Whereas most existing AI tools are trained on step five, predicting the final diagnostic label, Adler-Milstein et al would like to see novel diagnostic AI tools that capture the additional data generated during the wayfinding process and apply it during the iteration of steps one through three.
For instance, initial datasets could characterize the information-processing and decision nodes in the diagnostic process for common symptoms, and natural language processing could be applied to the clinical interview to suggest factors such as occupational exposures that could be relevant for determining an accurate diagnosis, the authors noted.
"New clinician-centric data are needed that capture clinicians' actions during the diagnostic process (e.g., what data clinicians typically review when evaluating a patient with low back pain) and the contextual factors that surround the clinician and patient during this process (e.g., team structure, patient volume)," Adler-Milstein et al wrote.
The authors recommended observing the current digital activities of clinicians during their wayfinding process to unveil where AI could be optimized to help clinicians find more accurate diagnostic pathways.
"The AI system, unconstrained by standard practices that make sense to clinicians, may uncover the safety and efficiency of alternative approaches that inform wayfinding guidance," they wrote.
AI could reach its full potential in diagnostics as a result from the shift away from end-label predictions, the authors noted.
"Diagnostic AI has not realized its potential to improve diagnostic performance because it has not focused on supporting the diagnostic journey," the authors wrote. "Knowing the direction of the pathway in a complex environment is important, but the essential decision is determining the next step."