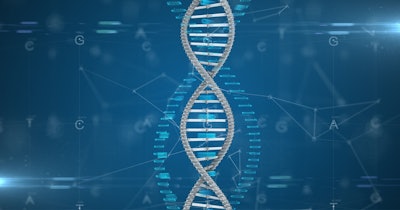
A gene-specific machine-learning approach is more efficient and effective than a disease-specific approach for predicting the pathogenicity of rare missense variants in in BRCA1 and BRCA2 genes, according to a recently published study.
BRCA1 and BRCA2 (BRCA1/2) genes are associated with an elevated risk of developing breast and ovarian cancers, with small germline variants of BRCA1/2 being one of the primary sources of such risk.
A team led by Kyu-Baek Hwang, a computer scientist at Soongsil University in Seoul, South Korea, investigated the efficacy of gene-specific supervised machine learning in predicting the pathogenicity of rare BRCA1/2 missense variants compared to the disease-specific approach.
Supervised machine learning has been widely adopted to develop computational tools for the pathogenicity prediction of variants, including rare missense ones. In previous studies, gene-specific predictors performed better than or comparable to genome-wide predictors. However, none of the studies have compared the gene-specific approach with the disease-specific approach, which researchers say is less specific but expected to have less variance.
The research team used 1,068 rare (gnomAD minor allele frequency [MAF] < 0.005) missense variants of 28 genes associated with hereditary cancers. The disease-specific training dataset included the gene-specific training dataset and was seven times larger.
“However, we observed that gene-specific training variants were sufficient to produce the optimal pathogenicity predictor if a suitable machine learning classifier was employed,” Hwang and colleagues wrote in Scientific Reports.
The researchers recommend the gene-specific method with the caveat that for genes with extremely low numbers of variants, a disease-specific approach may be more appropriate.